Understanding Business Data Analytics: A Comprehensive Overview
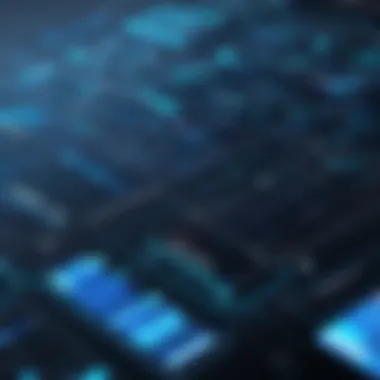
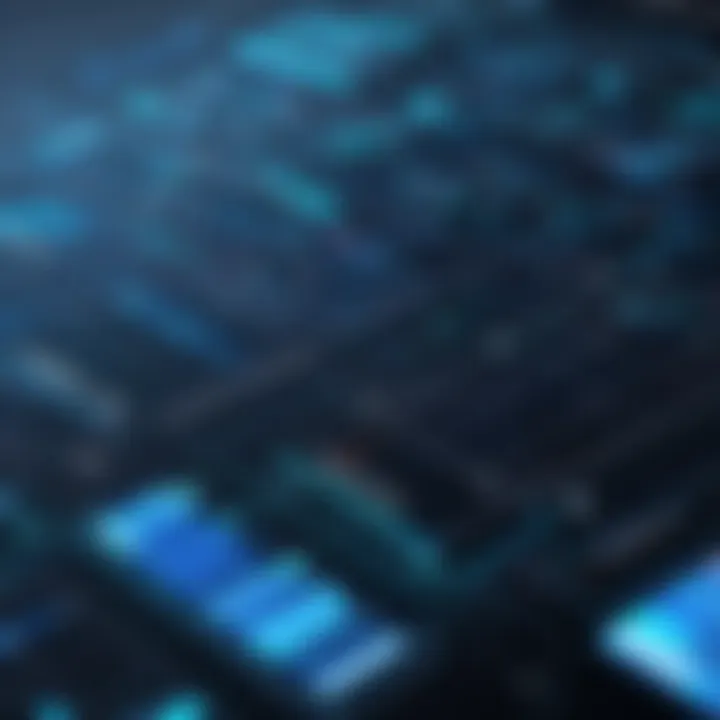
Intro
Business data analytics has emerged as a crucial component for organizations aiming to thrive in an increasingly data-driven world. This discipline encompasses a variety of techniques and tools that transform raw data into meaningful insights, empowering decision-makers to formulate strategies that enhance performance. A thorough understanding of business data analytics is essential as it not only drives operational improvements but also fosters innovation across various sectors.
Analyzing data enables organizations to identify trends, forecast outcomes, and optimize processes. This capability is vital for maintaining competitive advantages. Various methodologies, including descriptive, predictive, and prescriptive analytics, allow businesses to approach problems from multiple perspectives. This multifaceted approach ensures that data analytics goes beyond mere reporting, touching on strategic implications and practical applications.
In this overview, we will explore the features, advantages, and limitations of business data analytics in detail. We aim to provide readers with a comprehensive perspective on the topic, enabling business leaders and IT professionals to harness data effectively. By familiarizing ourselves with the tools and frameworks associated with data analysis, we can better navigate the complexities of today's business landscape.
Defining Business Data Analytics
In today's rapidly evolving business landscape, the concept of data analytics has emerged as a critical component for organizations seeking to leverage insights for strategic advantage. Defining business data analytics is not just about understanding its fundamental elements; it involves recognizing the significance of data in decision-making processes. This section aims to establish a foundation for understanding the various facets of business data analytics, which will be explored in detail throughout this article.
What is Business Data Analytics?
Business data analytics refers to the systematic computational analysis of data to gain insights and support decision-making within an organization. It encompasses a range of techniques, tools, and methods to process vast amounts of data collected from various sources.
The primary objective is to help businesses make informed decisions based on real-time data rather than intuition or guesswork. By analyzing past performance, organizations can forecast future trends and tailor their strategies accordingly. As the business world becomes increasingly dependent on data, understanding what business data analytics involves is paramount for all stakeholders.
Key Components of Business Data Analytics
To grasp the full scope of business data analytics, it is crucial to delve into its key components. Each element plays a significant role in converting raw data into actionable insights that drive organizational effectiveness.
Data Collection
Data Collection is the initial step in the analytics process. It involves gathering relevant data from a variety of sources, such as customer transactions, social media, and operational systems. This phase is vital as the quality and quantity of data directly affect the outcomes of the subsequent analysis.
A key characteristic of data collection is its broad range of methodologies, including surveys, web scraping, and automated data feeds. These diverse techniques allow businesses to capture a holistic view of relevant information. Its importance cannot be overstated; without comprehensive data gathering, the analytics process is built on a fragile foundation, leading to potential misinterpretations.
The unique feature of effective data collection lies in its ability to integrate different data types—structured and unstructured—providing a richer dataset for analysis. However, challenges can arise concerning data privacy and security, making it essential for organizations to adopt ethical and compliant practices throughout this phase.
Data Processing
Once data is collected, the next stage is Data Processing. This involves cleaning, organizing, and transforming raw data into a structured format that can be analyzed. It serves as a crucial bridge between data collection and analysis.
A significant characteristic of data processing is automation. Tools can streamline the process, enabling quicker data readiness for analysis. Automated workflows save time and reduce human error, which is a common issue during manual processing.
Moreover, effective data processing allows businesses to make use of real-time data. This can lead to timely insights that are essential for adaptive strategies. However, the complexity of processing data from disparate sources must not be underestimated. It requires robust infrastructure and skilled personnel to ensure accuracy and reliability in the processed data.
Data Interpretation
Data Interpretation is the final component that transforms processed data into meaningful insights. This phase involves analyzing the data to draw conclusions and make predictions about future trends. Skilled data analysts employ various statistical tools and visualization techniques to uncover patterns and relationships within the data.
A key characteristic of data interpretation is its reliance on analytical frameworks, such as regression analysis and clustering algorithms. These frameworks facilitate a systematic approach to deciphering complex data sets, making them accessible for decision-makers.
The unique advantage of effective data interpretation is the ability to craft narratives from data. Interpreting findings can enhance strategic foresight and drive business development. However, there is a risk of overfitting data—drawing conclusions that are too tailored to specific datasets without considering wider applicability. This emphasizes the importance of critical thinking and validation in analytics.
The Importance of Business Data Analytics
The significance of business data analytics cannot be overstated in today's fast-paced and data-driven environments. Organizations utilize analytics to gain insights that were previously unattainable. This facilitates informed decision-making, which is essential for sustained growth and competitive advantage. Every aspect of business, from marketing to finance, can benefit from a robust analytics strategy. This importance is reflected in a variety of elements.
Driving Strategic Decision-Making
Strategic decision-making embodies the crux of business success. With the help of analytics, executives can identify patterns and trends in data, leading to more effective strategies. For instance, market analysis based on customer data will allow companies to tailor their products according to consumer preferences. This leads to better customer satisfaction. Additionally, predictive analytics provides forecasts that help businesses navigate risks and opportunities.
Data-driven decision-making enhances clarity and reduces uncertainty. The reliance on subjective opinions diminishes as organizations ground their strategies in empirical evidence. This shift allows leaders to allocate resources more effectively. It becomes easier to pinpoint which strategies yield the best returns, allowing businesses to streamline operations and maximize profits. Incorporating analytics into the decision-making process fosters a culture of accountability, as teams can measure the effectiveness of their initiatives against established metrics.
Enhancing Operational Efficiency
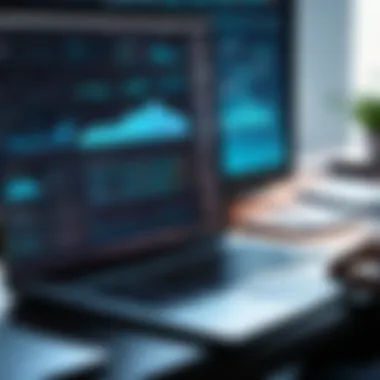
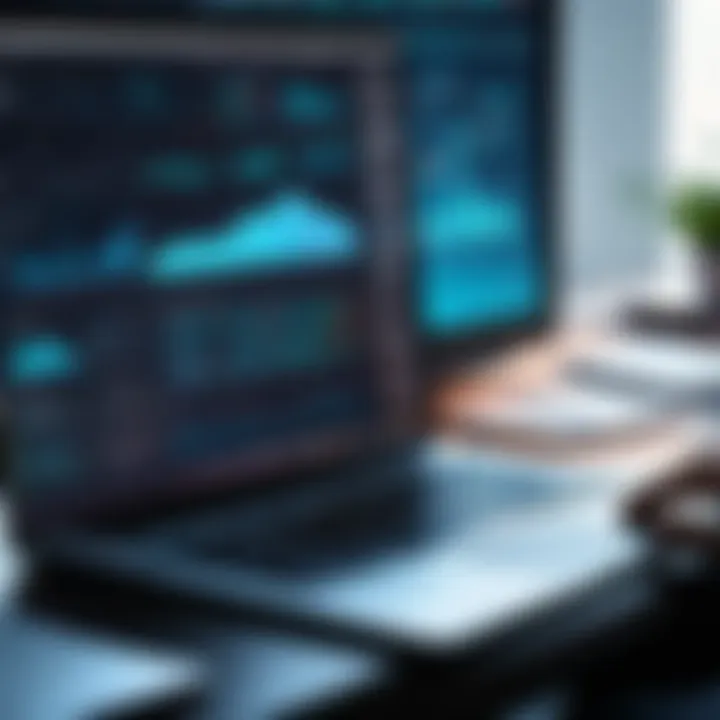
Operational efficiency is another crucial area where analytics plays a vital role. Organizations can harness analytics to evaluate their internal processes. This involves the analysis of workflow, resource allocation, and performance metrics. Identifying inefficiencies is easier with data insights, allowing companies to implement corrective measures promptly. This not only boosts productivity but also saves costs.
Moreover, real-time data analytics can offer insights into various operational aspects. For example, supply chain analytics monitors inventory levels and optimizes procurement schedules. This leads to reduced waste and better inventory management. Companies like Amazon rely heavily on analytics to streamline their operations.
In summary, the importance of business data analytics is evident in its ability to drive strategic decision-making while enhancing operational efficiency. Both aspects contribute significantly to a company's overall performance. As such, organizations that prioritize analytics are better positioned to adapt to market changes and outpace competitors.
Methodologies in Business Data Analytics
Methodologies in business data analytics play a critical role in ensuring that data is not just collected but is also analyzed effectively to support decision-making. These methodologies provide structured approaches to understanding data, making it easier for organizations to derive actionable insights. By utilizing specific methods, businesses can systematically identify trends, forecast future outcomes, and recommend steps that can be taken to improve performance. The integration of these methodologies into the analytical process significantly enhances strategic initiatives, operational efficiency, and overall business performance.
Descriptive Analytics
Descriptive analytics serves as the foundation of data analytics. It summarizes past data and provides insights into what has happened in a business. This type of analytics uses various methods to answer questions such as:
- What were the sales figures last quarter?
- How many customers visited the store last month?
Descriptive analytics employs statistical measures and visualization tools to present data in an understandable format. Common techniques include historical data analysis, data mining, and reporting dashboards. One significant benefit of descriptive analytics is its ability to offer a clear snapshot of performance over time, which can be invaluable in identifying patterns and trends. However, it is essential to recognize that while descriptive analytics provides insights into past performance, it does not predict future outcomes.
Predictive Analytics
Predictive analytics goes beyond mere description; it forecasts future events based on historical data and statistical algorithms. This involves using various statistical techniques such as regression analysis, time series analysis, and machine learning models. These methods help organizations anticipate outcomes such as customer behavior, market trends, and potential risks.
The utility of predictive analytics is especially noticeable in sectors like retail, finance, and healthcare. For instance, retailers can utilize predictive models to optimize inventory by forecasting demand for products. On the other hand, financial institutions leverage predictive analytics to assess the risk involved in loan applications. Despite its numerous advantages, it is crucial for organizations to constantly update their models to reflect changing market conditions. Predictive analytics can be powerful, yet its accuracy hinges on the quality of underlying data and assumptions made during modeling.
Prescriptive Analytics
Prescriptive analytics takes analytics a step further by providing actionable recommendations based on insights derived from data. It answers the question of how to handle future scenarios. This methodology combines data, statistical algorithms, and business rules to suggest courses of action. It often employs optimization and simulation algorithms to evaluate the potential outcomes of different decisions.
For example, in supply chain management, prescriptive analytics can identify the most efficient routes for delivery or determine how to allocate resources effectively. It helps businesses not only understand what happened and what is likely to happen but also suggests how to influence future outcomes positively. However, while prescriptive analytics can significantly impact decision-making, it requires robust data inputs and careful interpretation to implement the recommended actions successfully.
"Organizations that leverage methodologies like predictive and prescriptive analytics can create a sustainable competitive advantage, preparing them for future challenges."
Employing these methodologies enables businesses to extract the most value from their data by aligning analytical practices with strategic goals. As such, understanding these methodologies is essential for professionals seeking to enhance their analytical capabilities.
Tools and Technologies for Data Analytics
In today’s world, the significance of tools and technologies for data analytics cannot be overstated. For organizations striving to remain competitive, the ability to leverage data effectively is paramount. These tools enable businesses to extract meaningful insights from data, allowing for informed decision-making that can drive growth and efficiency. This section will explore various critical tools that form the backbone of data analytics in business context.
Data Visualization Tools
Data visualization tools play a crucial role in translating complex data sets into understandable formats. They allow users to create visual representations such as charts, graphs, and dashboards that summarize large volumes of data concisely.
Benefits of using data visualization tools include:
- Enhanced Understanding: Visual formats facilitate quicker comprehension and recognition of patterns.
- Improved Decision-Making: Clear visual insights support more effective decisions based on data.
- Stakeholder Communication: Visuals simplify information sharing with stakeholders who may not have technical backgrounds.
Popular data visualization tools include Tableau, Power BI, and Google Data Studio. These tools have features that cater to different needs, whether real-time data updates or integration with existing databases.
"A great data visualization tool does more than just present data; it answers questions and prompts new inquiries."
Statistical Analysis Software
Statistical analysis software is indispensable when it comes to conducting rigorous data analyses. These tools provide functionalities to perform various statistical tests and models, making it possible to draw conclusions from data.
Some benefits include:
- Robust Analytical Capabilities: Support for complex calculations and statistical validations.
- Versatile Applications: Used across different disciplines from finance to healthcare for various analytical needs.
- User-Friendly Interfaces: Many modern statistical packages provide straightforward interfaces, thus lowering the barrier for entry.
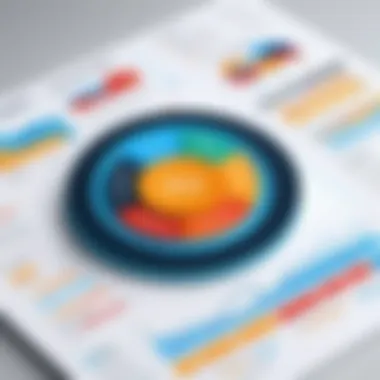
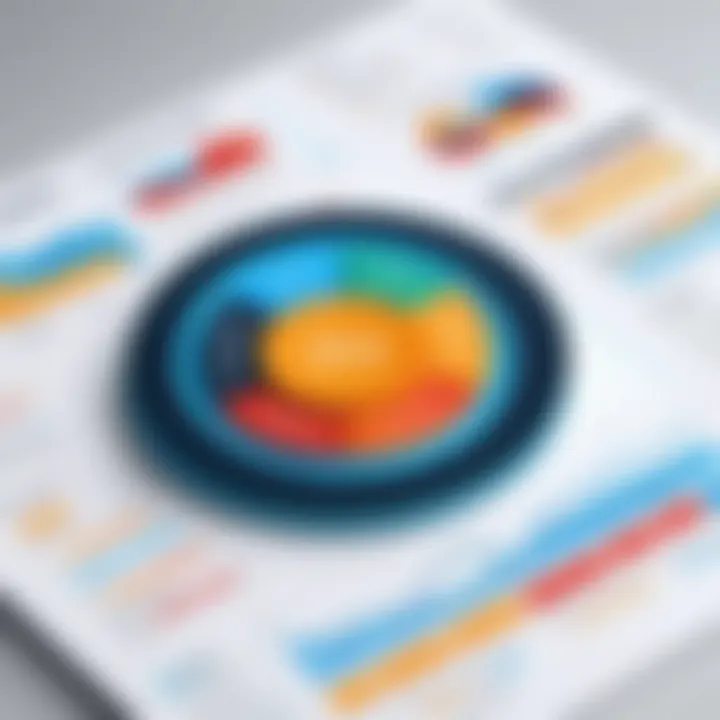
Notable software applications in this domain include R, SAS, and SPSS. Each offers unique capabilities, catering to both novice and advanced users.
Data Mining Techniques
Data mining techniques are essential for discovering patterns or relationships within large data sets. Companies can employ these techniques to identify trends, enhance customer experiences, and predict future behaviors.
Key advantages of data mining include:
- Trend Identification: Understanding trends helps in strategizing marketing and product development.
- Risk Management: Predictive analytics can identify potential risks before they impact the business.
- Customer Insights: Deep dives into customer data can inform targeted marketing efforts.
Common data mining techniques include clustering, classification, and regression. Mastery of these techniques enhances an organization’s analytical toolkit, leading to better outcomes.
In summary, tools and technologies for data analytics are essential for modern businesses. They empower organizations to make informed decisions based on accurate data analysis. By implementing the right combination of data visualization tools, statistical analysis software, and data mining techniques, companies can extract valuable insights, driving their strategies forward.
Implementation Challenges in Business Data Analytics
Implementing business data analytics is vital for organizations striving to leverage insights for competitive advantage. However, several challenges arise during this process. Addressing these obstacles is essential to ensure that analytics initiatives are successful and deliver value. This section explores key issues that organizations might face when implementing data analytics and provides insights into navigating these challenges effectively.
Data Quality Issues
One of the most significant challenges in business data analytics is ensuring data quality. Poor-quality data can lead to incorrect insights and misguided decision-making. Organizations often struggle with data that is inconsistent, incomplete, or outdated. Such issues can stem from multiple sources, including manual data entry errors, integration issues, and lack of standardized procedures.
To counteract data quality issues, businesses should prioritize the establishment of robust data governance frameworks. This framework defines the procedures for data collection, storage, and usage. Regular data cleansing is critical to maintain integrity and accuracy. By ensuring that data is reliable, businesses can improve the effectiveness of their analytics efforts.
"Accurate data is the foundation of reliable analytics."
Integration with Existing Systems
Another challenge is the integration of data analytics tools with existing systems. Many organizations have legacy systems that may not be compatible with newer analytics solutions. This can create data silos, where information is trapped in specific systems and not readily accessible for analysis. As a result, the full potential of data analytics may be underutilized.
To facilitate effective integration, businesses should assess their current IT infrastructure. They need to identify areas where compatibility issues may arise. Adopting an agile integration approach can help organizations bridge the gap between legacy and modern systems. Ensuring that analytics tools can communicate seamlessly with various data sources is essential for obtaining a holistic view of organizational data. This approach enhances the overall efficiency and effectiveness of data analytics initiatives and enables informed decision-making.
Ethical Considerations in Data Analytics
In the ever-evolving landscape of business data analytics, ethical considerations play a pivotal role. As organizations collect, analyze, and interpret vast amounts of data, they must navigate the complex interplay between data utilization and ethical integrity. This section highlights the significance of ethical considerations in data analytics, focusing on two primary elements: data privacy and security, and transparency in analytics practices.
Data Privacy and Security
Data privacy is an essential aspect of ethical data analytics. Organizations must handle personal data responsibly to protect individuals’ rights and trust. As they collect sensitive information, such as customer details and behavioral patterns, a thorough understanding of data protection regulations, like the General Data Protection Regulation (GDPR), is crucial. These regulations impose strict guidelines on data usage, ensuring individuals have control over their personal information.
Moreover, ensuring security measures are in place is of utmost importance. Data breaches can lead to significant financial and reputational damage. Adopting robust cybersecurity frameworks and encrypting sensitive data are two key strategies organizations can employ to mitigate risks. Regular audits of security protocols can also help to identify vulnerabilities and strengthen defenses against potential threats.
Data privacy is not merely a compliance issue; it is a cornerstone of consumer trust and long-term business success.
Maintaining privacy not only enhances loyalty but also improves customer relationships. Transparent communication with consumers regarding how their data is used fosters an environment of trust.
Transparency in Analytics Practices
Transparency in analytics practices is another critical component of ethical data analytics. Organizations should provide clear insights into how data is collected, processed, and analyzed. This openness builds credibility and reassures stakeholders that the data is being used responsibly.
Transparency involves creating clear documentation on methodologies used in data analysis. Organizations must ensure that their data analytics processes are easily understandable, enabling stakeholders to engage with the findings meaningfully. Furthermore, being transparent about potential biases in algorithms can help to avoid misunderstandings and ethical dilemmas.
Organizations should also encourage feedback from customers regarding their data practices. This will not only improve existing practices but also align the organization with customer expectations.
The Future of Business Data Analytics
The future of business data analytics presents an exciting landscape full of potential for organizations looking to harness insights from data. As the digital era continues to evolve, businesses are faced with vast volumes of data that can lead to transformative changes in decision-making and strategy. Thus, understanding the future trends in data analytics is essential for leaders who seek to leverage data for competitive advantage.

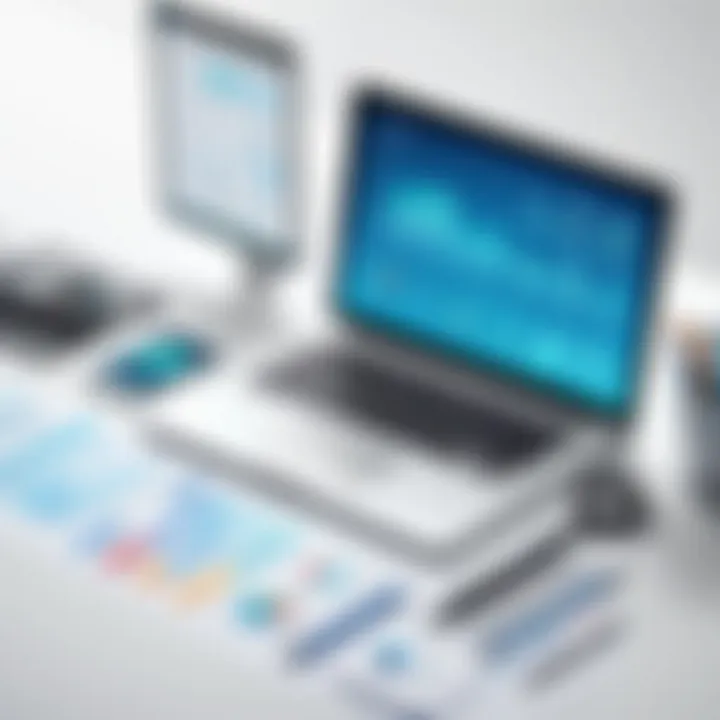
Emerging Trends
Artificial Intelligence
Artificial intelligence plays a significant role in shaping the future of business data analytics. The key aspect of AI is its capability to mimic human intelligence to process information and learn from data. This is a beneficial choice for organizations because it can drive efficiency in analyzing large datasets at unprecedented speeds.
A unique feature of AI is its ability to visualize patterns and trends that might be overlooked in manual analyses. Implementing AI algorithms can reduce the time spent on data processing and increase the accuracy of forecasting outcomes. However, challenges exist, including ethical considerations and potential biases in data interpretation. These concerns must be adequately managed to ensure the reliability of AI-driven analytics.
"The implementation of AI in data analytics is not just a trend, but a foundational shift in how businesses operate and make decisions."
Machine Learning
Machine learning is another pivotal area contributing to the future of business data analytics. Its hallmark characteristic is the ability to learn from existing data to make predictions about future events. This makes machine learning an ideal tool for organizations looking to enhance their predictive analytics capabilities.
In this context, machine learning can analyze past consumer behavior to provide insights into future purchasing patterns. The unique feature of machine learning is its adaptability; it can improve over time as more data becomes available. Despite its advantages, such as scalability and versatility, organizations must be cautious with overfitting — a situation where a model is too closely aligned to the training data, leading to poor performance in real-world application.
The Role of Big Data
Big data continues to be a driving force behind advancements in business data analytics. With the exponential growth of data generated daily, organizations must not only store it but also understand how to derive meaningful insights. The role of big data manifests in its ability to inform strategies across various sectors, from marketing to supply chain management.
The significance of big data lies in its volume, velocity, and variety. This combination can yield comprehensive analyses that inform critical business decisions. However, tapping into big data requires sophisticated tools and expertise to ensure that the data is accurate, relevant, and actionable. As companies adopt big data strategies, they will need to balance the opportunities it presents with the risks associated with data privacy and security.
Epilogue
The conclusion of this comprehensive overview on business data analytics serves as an important culmination of discussed themes and insights. Understanding the significance of data analytics is essential for modern organizations. It not only illuminates key findings but also articulates the implications that arise from these insights. Practitioners can utilize the concepts covered to inform their strategies and foster better decision-making processes.
In summary, it is critical to recognize how business data analytics enables informed decision-making. Organizations that embrace data-driven practices are often better equipped to navigate uncertainties and optimize their operations. The synthesis of results leads to actionable insights that can enhance business performance in various facets.
Summary of Key Insights
The article presents several key insights that resonate across various business contexts. For instance, it is apparent that data collection, processing, and interpretation are vital components of the analytics process. Furthermore, methodologies such as descriptive, predictive, and prescriptive analytics create a robust framework for analyzing business data.
Key insights include:
- Data-Driven Decision Making: The necessity of basing decisions on data rather than intuition alone can lead to more effective outcomes.
- Operational Efficiency: Organizations that leverage analytics can streamline processes and enhance productivity.
- Emerging Technologies: The integration of artificial intelligence and machine learning in analytics provides a competitive edge.
- Data Quality and Ethics: High-quality data is paramount, and ethical considerations in data use remain critical.
Implications for Practitioners
For practitioners, understanding business data analytics carries substantial implications for their roles and responsibilities. It mandates a shift towards a culture that values data at every level. Embracing analytics requires an investment in training and tools that facilitate better analysis capabilities.
Key implications consist of:
- Strategic Investment: Businesses may need to allocate resources towards acquiring advanced analytics tools and skilled personnel.
- Continuous Learning: Ongoing education and adaptation to new methodologies are necessary for maintaining relevance in an evolving landscape.
- Collaboration Across Departments: Data analytics should foster collaboration among departments, ensuring that insights are shared and utilized across the organization.
- Heightened Focus on Data Ethics: As reliance on data grows, adhering to ethical practices will protect both the organization and its stakeholders.
The importance of conclusion in relation to business data analytics is evident, as it brings together essential themes crucial for understanding and leveraging analytics in a corporate environment.
Importance of References
References are crucial in establishing a framework for the assertions made within any analytical discussion. They serve several distinct purposes:
- Credibility: By citing reputable sources, the article gains authority. Readers are more likely to trust information that is corroborated by expert voices in the field.
- Context: References provide context by situating the discussion within the larger body of knowledge. This helps readers to see how the insights from this article fit into ongoing dialogues in the industry.
- Resources for Further Study: For those interested in delving deeper, references act as a gateway to additional literature. They enable readers to explore complex topics in greater detail.
Examples of Useful References
The following resources can provide further insights related to business data analytics:
- Wikipedia: The Wikipedia page on data analytics offers a broad overview of the field, including definitions and types of analytics.
- Britannica: For a more scholarly treatment, the Encyclopedia Britannica on business analytics provides historical context and developments in the discipline.
- Reddit: Engage with a community of professionals and enthusiasts discussing real-time applications and experiences in data analytics on platforms like Reddit.
- Facebook: Various groups on Facebook allow for networking and collaboration among data analytics practitioners.
"In the quest for insights, never underestimate the power of a well-placed reference."
Considerations about References
In crafting references for business data analytics, it is important to:
- Assess the Authority: Verify that sources come from respected experts or institutions.
- Evaluate Recency: Ensure that the information is up-to-date, particularly in a fast-moving field like technology.
- Diversity of Sources: Use a variety of references to present balanced viewpoints on the subject.